February 23, 2022
A Question-Answering model that answers EVERYTHING
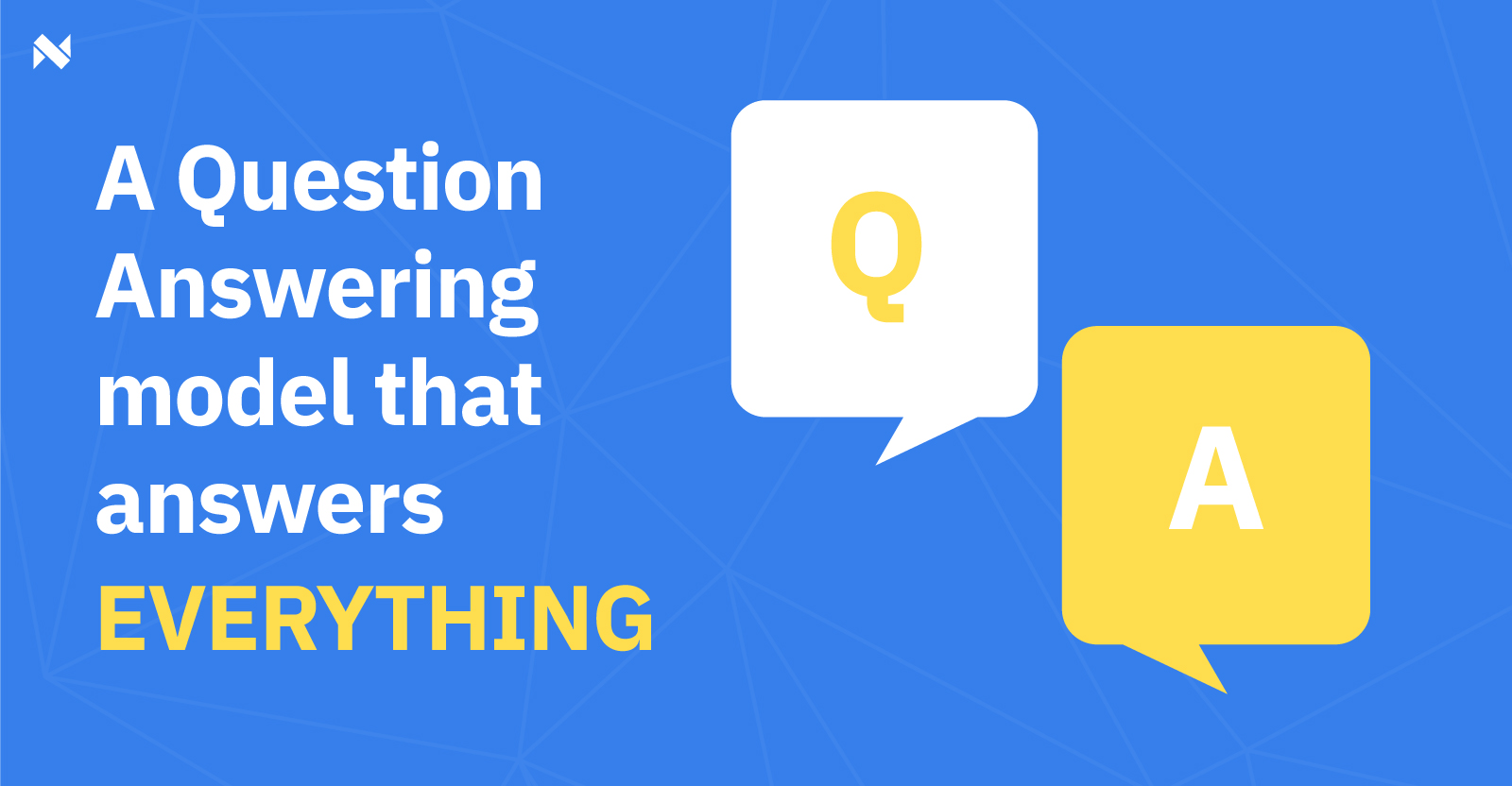
By Sofía Sánchez González
Although NLP technology has come a long way, up until now Question-Answering models have been limited to the text with which we train them. If text isn’t provided, it would be like having a roadmap without street signs or landmarks; there would be no context. But now everything has changed with a new open domain Question-Answering model that answers EVERYTHING.
Let’s start from the beginning:
What is Question-Answering?
Question-Answering (QA) models are simply information retrieval systems that search for answers to queries posed by humans and automatically communicate results in a natural language. How is this achieved? By training them with documents such as a handful of Wikipedia pages, or in the case of a business, internal reports and forms.
Here’s an example that explains a situation we might encounter here at Narrativa: Let’s imagine a report on your company’s financial results over the last 15 years; it’s more than 50 pages with all kinds of context and details about the movements and steps taken by the company, such as mergers and sales for instance. And for simplicity’s sake, you want to know the year that a certain merger occurred.
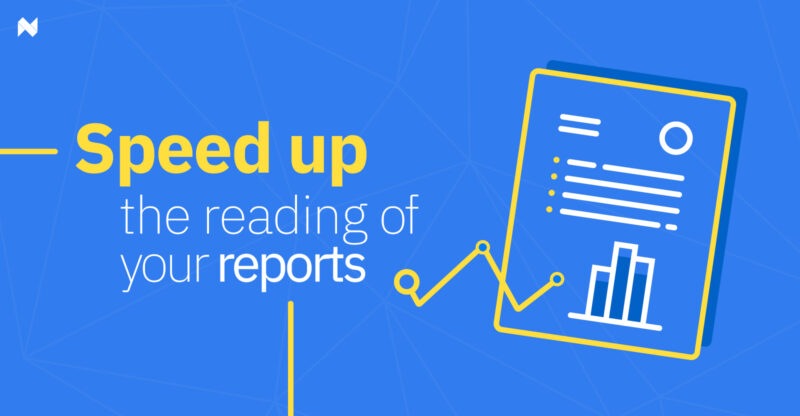
Question-Answering (QA) models are simply information retrieval systems that search for answers
How do you get the info you need fast? Our QA model! We pass the report to our model and ask the question “In what year did the merger with X company take place?” The model gives us the answer quicker than you can snap your fingers and saves you an enormous amount of time manually thumbing through pages looking for keywords with your own eyes. Indexes, glossaries and footnotes, oh my!
Short summary so far: Reader models
So far, Reader models have been deeply developed. If a question is asked and the answer is within context, the model tells me where the answer begins and ends. They are extractive. Conversely, if the answer is not in context, the model doesn’t produce anything.
Unity is strength: two QA models for the price of one
In the example above about the financial report, the model gave us the answer we wanted, sure. But what if it’s necessary to search more than a single report? What if we want to ask something random? Well, we also have the answer to that too: use a hybrid of two models to get the best result. Unity is strength in artificial intelligence.
Open Domain QA: retriever + reader models
Unfortunately, we are not referring to golden retrievers (we know, we’re dog lovers too though!). However with this type of retriever, information is attained even if the question isn’t formulated exactly as the text is written. The reader model indicates an approximation whereas the retriever takes you to the exact spot where the information is located. Both models work in tandem to give us the answer. And of course, we can save a lot of time if we know where the answers to our questions are! You can find more insight about this topic in this article.
Big companies already use them
At Google we can already see examples of this model, since the search range is greatly reduced.
Before their introduction, Google searched “manually” among its indexed content. But now everything is much easier. Here, we searched for “How to take a screenshot in Windows” and it directly identifies what we’re looking for.
For any company that has endless droves of documents or text-based reports, this solution can be invaluable. Or if, for example, we are a sports media outlet, we can ask:
Who was Real Madrid’s best scorer last season?
You can already imagine how many articles talk about this topic on any given sports website that covers Real Madrid, but with the confluence of both models you can have your answer instantaneously. Now if only we could get our hands on some golden retriever puppies to play with that fast as well…
What about regulatory submissions?
No, the model is not optimized to answer regulatory submission inquiries related to clinical study reports (CSRs) or other regulatory documents. Why? Because this model is not solely equipped to serve the needs of the Life Sciences industry. Therefore, it cannot be used to automate or answer questions related to patient safety narratives or Tables, Lists, and Figures (TLFs) since these documents consist of complex information derived from millions of patient data points.
Is there an alternative for regulatory submissions? Yes, Narrativa has worked with industry leaders and medical writers for years to develop and enhance a suite of solutions for the Life Sciences industry. Currently, our solutions involve the automation of patient safety narratives and TLFs to help expedite the process of CSR creation, giving room for medical writers to allocate their time and efforts toward more critical tasks that require significant human input.
About Narrativa
Narrativa is an internationally recognized business intelligence company that uses its artificial intelligence and machine learning platform to build and deploy natural language content solutions for enterprises. Its proprietary technology suite, consisting of data extraction, data analysis, natural language processing (NLP) and natural language generation (NLG) tools, all work together seamlessly to power a lineup of smart content creation, reporting automation and process optimization products.
Contact us to learn more about our solutions!
Share