December 21, 2020
What is GPT-3?
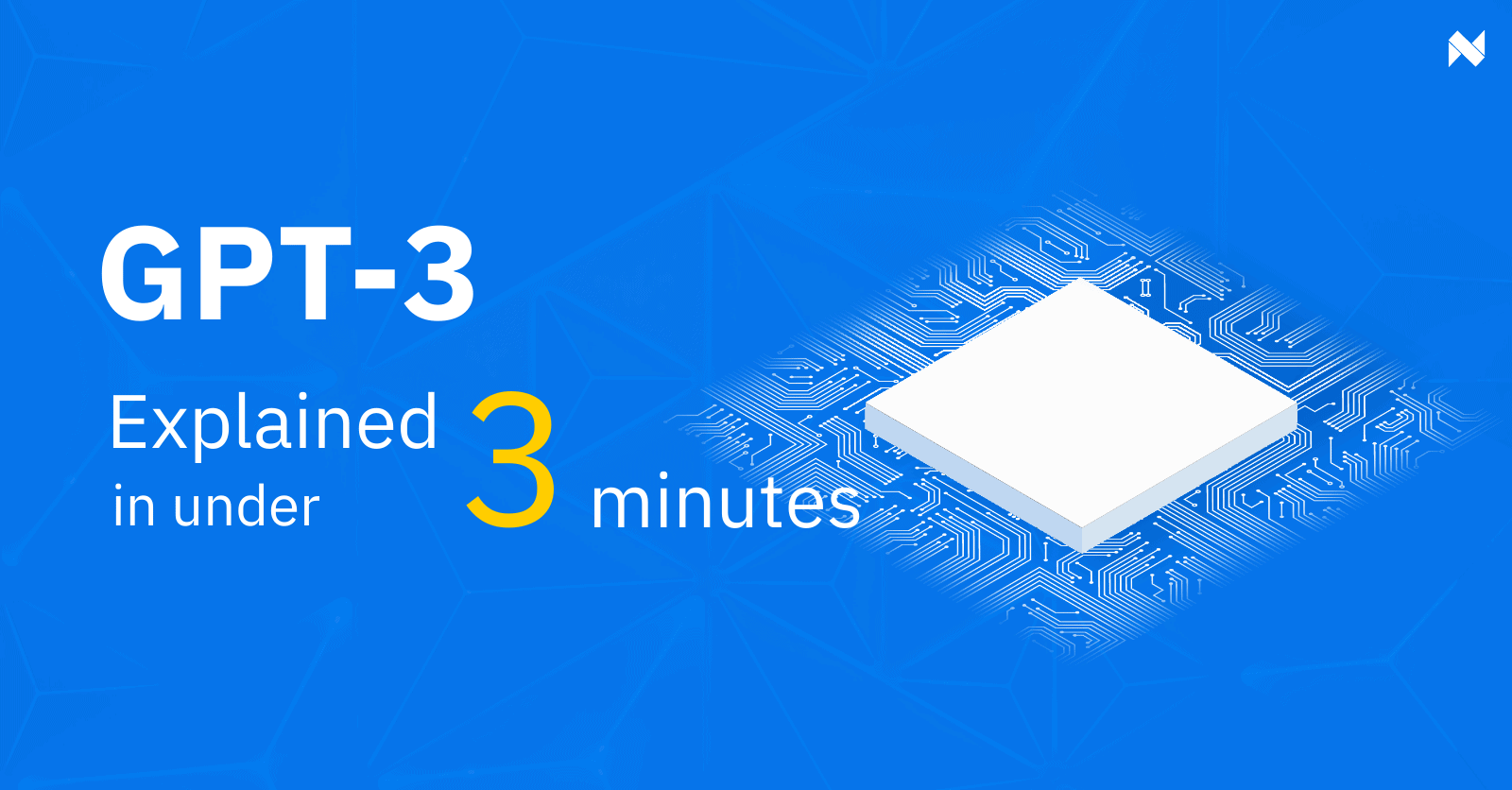
By Narrativa Staff
Since its launch midway through this year, we have not stopped hearing about the GPT-3 model. Major news media in the fields of science and programming from across the globe have lost the plot with the launch of this new technology, which for many represents a “before and after” in the realm of artificial intelligence.
So what is it all about? Is it really as impressive as they say? Is it all hyperbole or is everything they say true? What are its flaws or limitations? Let us explain it to you.
Better reading comprehension than a human being
GPT-3 is a language model developed by OpenAI (an initiative promoted by Elon Musk) that learns from existing text and can provide different ways of finishing a sentence (similar to predictive text). But GPT-3 doesn’t come like that out of the box; it needs to be trained with thousands and thousands of examples, something that has only been done in English so far.
However, once trained, it can save you a lot of time, providing linguistic richness and variability along with perfect grammar. In addition, it is capable of answering a wide variety of questions.
So, how does it do it?
- It uses 175 billion parameters.
- It has been trained with 500 billion words.
- Its reading comprehension is superior to that of the average human.
- Almost $5 million USD was invested in its training.
To give you an idea of the importance of this model for the world of artificial intelligence, let us just say that GPT-3 is 100 times bigger than its predecessor, model GPT-2.
Building this gigantic neural network has taken considerable expense: no less than 12 million dollars, and even then it still has its controversial (and weak) points.
Nobody’s perfect: complex and inaccessible
With all this data, how can many doubt its impact on the world of Natural Language Generation (NLG)?
1. Its level of complexity is huge. GPT-3 is so expansive (175 billion parameters) that it is not possible to make small adjustments to adapt the text to our needs (what in Natural Language Processing is known as fine-tuning).
GPT-3 simply learns something by heart but cannot extrapolate. In addition, the model may have biases. Let’s not forget that the text used to train GPT-3 has been written by humans, making it difficult to control the outcome.
2. It is capable of generating fake news. However, if your intention is to train the model to generate news about the Earth being flat, you may not succeed.
GPT-3 will generate a news story from the text already written about the Earth, so it is highly unlikely that it will come to this conclusion. It is capable of writing a coherent article, but this may not be exactly what you want (whether your intentions are good or bad).
3. It isn’t very accessible. OpenAI have granted limited access to a privileged few (including Narrativa). So, who gets full access to the model and all the data needed to train it? Unfortunately, only the biggest companies. Very, very few.
Some companies are already building their artificial intelligence products around GPT-3, but clearly not everyone can afford the cost.
Future of the model
It is very difficult to surpass the limits that GPT-3 has achieved, simply because there isn’t any more written text in English to provide further training. This means it can’t continue to grow.
So how can the model evolve? GPT-3 would have to be able to understand the world, not just one language. When a child is told what a car is, the next time they see one they understand what it is (even if it is a different model). For the GPT-3 model to understand what a car is, it would have to see thousands of images of different models.
For now, that is a skill only humans possess. The question is: for how long?
Can GPT-3 be used for regulatory submissions?
No, because regulatory submissions have a level of complexity and multiple inputs that GPT-3 solely cannot comprehend. For example, writing a clinical study report (CSR) requires using documents like patient narratives and Tables, Lists, and Figures (TLFs). Creating such files requires connecting millions of data points involving thousands of patients. GPT-3 is not optimized to perform such tasks.
We at Narrativa have worked with our partners over the past several years on automating regulatory submissions that help them expedite the CSR submission process in a fast, cost-efficient manner. Also, our TLF and patient narrative automation solutions have helped save our partners’ medical writing teams an inordinate amount of time and effort.
Share